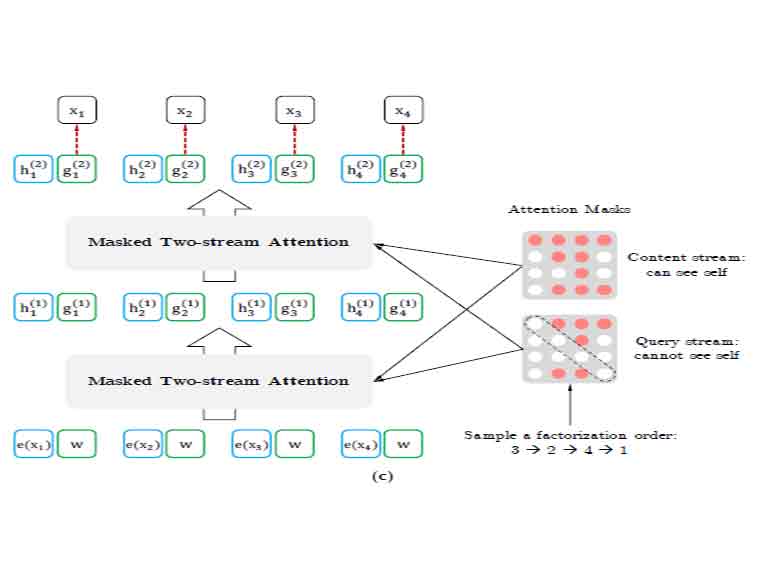
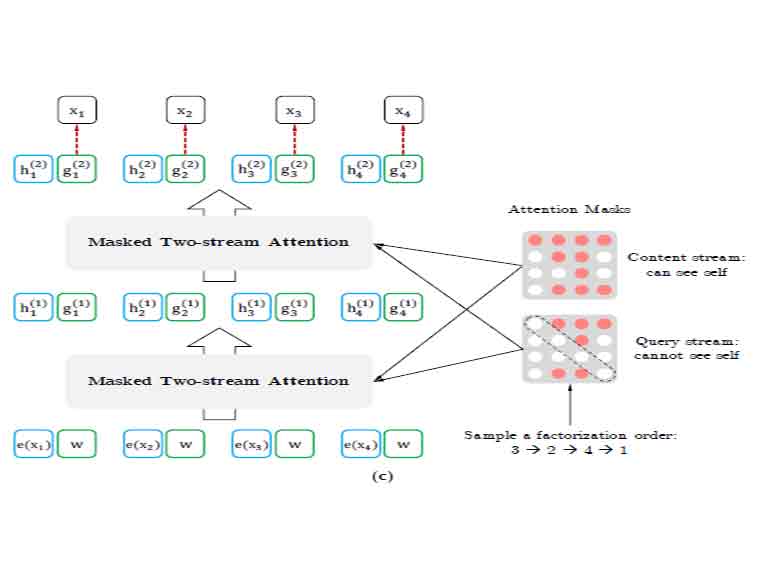
Natural Language Processing (NLP) stands at the forefront of modern artificial intelligence, bridging the gap between human communication and machine understanding. As our reliance on digital communication grows, so does the need for sophisticated language models capable of grasping the nuances and intricacies of human language. In this dynamic landscape, XLNet emerges as a pivotal player, ushering in a new era of NLP capabilities.
The Evolution of Natural Language Processing
To grasp the profound impact of XLNet, one must embark on a journey through the evolutionary tapestry of Natural Language Processing (NLP). Originating in the rudimentary days of rule-based systems, where algorithms adhered to predefined linguistic rules, NLP has metamorphosed into a dynamic discipline. The current epoch is marked by a machine learning renaissance, with a pronounced shift towards data-driven methodologies, notably harnessing the potency of deep learning. This transformative trajectory has catapulted NLP to unprecedented heights, fostering a landscape where models like XLNet emerge as pioneers, pushing the boundaries of language understanding and representation.
The Rise of Transformer Models
The paradigm shift in Natural Language Processing (NLP) is intricately tied to the revolutionary emergence of transformer models. At the nucleus of this transformation stands the transformer architecture, a brainchild of Vaswani et al. unveiled in the seminal paper “Attention is All You Need.” Departing from the conventions of recurrent and convolutional architectures, transformers represent a pioneering stride in processing information. Through the strategic incorporation of attention mechanisms, transformers process input data in parallel, unlocking unprecedented efficiency in capturing long-range dependencies and intricate relationships within language. This departure from sequential processing not only marks a departure from traditional limitations but also paves the way for a more nuanced, adaptable, and contextually aware approach to language understanding. The architectural alchemy of transformers has ushered in an era where the orchestration of attention becomes the linchpin, fostering a symphony of linguistic comprehension that transcends the boundaries set by earlier NLP models.
A Paradigm Shift in Language Modeling
In the diverse landscape of transformer-based models, XLNet emerges as a beacon of innovation, distinctly setting itself apart from its counterparts. In the backdrop of models like BERT (Bidirectional Encoder Representations from Transformers) and GPT (Generative Pre-trained Transformer), both of which had made substantial contributions to the field, XLNet introduces a paradigm-shifting methodology—permutation language modeling. This novel approach marks a departure from the autoregressive and autoencoding methods that were the hallmark of its predecessors. By leveraging permutation language modeling, XLNet transcends the constraints of unidirectional processing, paving the way for a more nuanced and comprehensive understanding of contextual relationships within language. This departure from the conventional methods not only underscores XLNet’s commitment to pushing the boundaries of language representation but also positions it as a trailblazer in the continual evolution of transformer-based models in the realm of Natural Language Processing.
The Autoregressive vs. Autoencoding Conundrum
To truly appreciate the innovative prowess of XLNet, it is imperative to delve into the fundamental nature of language models, specifically examining the autoregressive and autoencoding paradigms that have defined the landscape of Natural Language Processing (NLP). Autoregressive models, typified by the likes of GPT (Generative Pre-trained Transformer), operate by generating sequences in a step-by-step fashion. These models predict the subsequent word in a sequence based on the preceding tokens, gradually assembling a coherent output. GPT’s ability to generate contextually rich and coherent text has been a hallmark of autoregressive modeling, showcasing its proficiency in capturing the sequential dependencies within language.
On the other hand, autoencoding models, with BERT (Bidirectional Encoder Representations from Transformers) as a prime exemplar, follow a different trajectory. In the autoencoding paradigm, certain words within a sentence are strategically masked, and the model is tasked with reconstructing the original sequence. This bidirectional approach allows BERT to consider contextual information from both the left and right sides of a given word, transcending the unidirectional limitations inherent in autoregressive models. BERT’s success in capturing bidirectional context has been instrumental in a myriad of NLP tasks, from sentiment analysis to question answering.
However, despite the strides made by autoregressive and autoencoding models, both approaches harbor inherent limitations. Autoregressive models face challenges in parallelizing computations due to their sequential nature, leading to slower processing times and increased computational demands. Autoencoding models, while adept at capturing bidirectional context, may struggle with tasks that require generating coherent sequences, as they lack the autoregressive generation process’s finesse.
Enter XLNet, a paradigm-shifter in the NLP domain. XLNet introduces a novel approach known as permutation language modeling, transcending the binary constraints of autoregressive and autoencoding methods. This innovative methodology combines the strengths of both paradigms while mitigating their weaknesses. XLNet considers all possible permutations of a sequence during training, thereby incorporating bidirectional context and overcoming the computational bottlenecks associated with autoregressive models. This departure from the norm propels XLNet into the forefront of transformer-based models, offering a nuanced and adaptive solution to the challenges posed by traditional language modeling paradigms.
Permutation Language Modeling Contextual Relationships
At the heart of XLNet’s revolutionary architecture lies the concept of permutation language modeling—a distinctive paradigm that propels XLNet beyond the confines of traditional autoregressive and autoencoding models. This groundbreaking approach intertwines the strengths of both methodologies, marking a departure from conventional constraints. Unlike its predecessors, XLNet introduces bidirectional context by meticulously considering every possible permutation of a sequence during training. This meticulous exploration of permutations enables XLNet to capture dependencies emanating from both forward and backward directions, fostering a holistic understanding of contextual relationships within language. This innovative strategy not only overcomes the inherent limitations of autoregressive and autoencoding models but elevates XLNet to a realm where it excels in gleaning richer, more nuanced contextual information from input data. In the intricate dance of language processing, permutation language modeling positions XLNet as a trailblazer, seamlessly navigating bidirectional dependencies to decode the complexities inherent in diverse linguistic landscapes.
The Complexity of XLNet Architecture
As we embark on our journey to unravel XLNet’s intricacies, a deep dive into its architecture is paramount. Understanding how XLNet processes information bidirectionally, leveraging permutations, and navigating the challenges associated with this approach lays the foundation for comprehending its transformative impact on NLP.
In the subsequent sections of this comprehensive guide, we will explore the architecture of XLNet, the nuances of permutation language modeling, its pre-training and fine-tuning mechanisms, and its applications across diverse domains. Through this exploration, we aim to provide a holistic understanding of XLNet, shedding light on its strengths, challenges, and the exciting possibilities it unlocks in the realm of natural language processing. Let us embark on this enlightening journey into the heart of XLNet, where innovation converges with language to shape the future of AI.