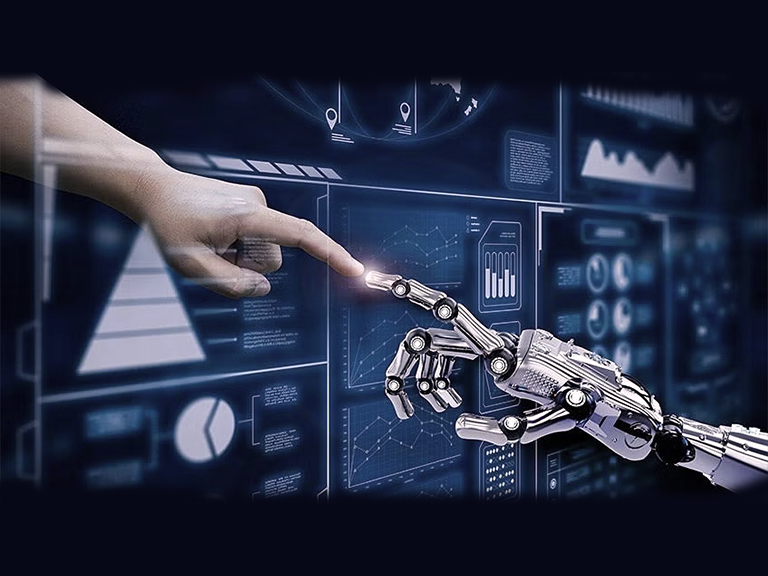

Machine Learning (ML) stands at the forefront of technological innovation, reshaping the landscape of computer science and artificial intelligence. In this comprehensive exploration, we will navigate through the intricate web of Machine Learning, unraveling its core concepts, and understanding the mechanisms that fuel its transformative power. By the end of this expansive article, you will possess a profound comprehension of the principles governing Machine Learning and its diverse applications.
Understanding Machine Learning
Definition and Objectives
Machine Learning is a dynamic field that empowers computers to learn and make intelligent decisions without explicit programming. At its core, the primary objective of ML is to develop algorithms that enable systems to improve their performance over time by learning from data. This learning process involves recognizing patterns, making predictions, and adapting to new information.
Learning Paradigms
There are three fundamental learning paradigms in Machine Learning:
Supervised Learning: In this paradigm, the algorithm is trained on a labeled dataset, where each input is associated with the correct output. Common algorithms include linear regression, logistic regression, support vector machines, decision trees, and random forests.
Unsupervised Learning: Here, the algorithm explores patterns and structures within the data without explicit guidance. Clustering algorithms like k-means and hierarchical clustering, dimensionality reduction techniques such as PCA and t-SNE, and anomaly detection methods fall under unsupervised learning.
Reinforcement Learning: This involves an agent learning by interacting with an environment and receiving feedback in the form of rewards or penalties. Markov Decision Processes, Q-learning, and policy gradients are common reinforcement learning approaches.
Key Components
Machine Learning involves four key components:
Data: The raw material that fuels the learning process. Quality data is crucial for training accurate models.
Features: Characteristics or attributes of the data that the algorithm uses to make predictions.
Models: The algorithms that learn from data and make predictions.
Algorithms: The set of rules or procedures that the model follows to learn from data and make predictions.
Labeled Training Data
In supervised learning, labeled training data is essential. Labels are the correct outputs corresponding to the input data, guiding the algorithm during training. The accuracy of the model heavily relies on the quality and quantity of labeled data.
Feature Extraction and Engineering
Transforming raw data into meaningful features is a critical step. Feature extraction involves selecting relevant information, while feature engineering involves creating new features to enhance model performance.
The Workflow of Machine Learning
Data Preprocessing
Before feeding data into a model, preprocessing steps are crucial. Handling missing values, normalization, and feature scaling ensure that the data is in a suitable form for training.
Data Splitting
Dividing the dataset into training, validation, and testing sets is vital to assess the model’s performance. The training set is used for training the model, the validation set for fine-tuning, and the testing set for evaluating the final performance.
Model Selection and Training
Choosing an appropriate model or algorithm depends on the nature of the problem. The model is then trained using optimization techniques like gradient descent to minimize the error or loss function.
Model Evaluation
The performance of the trained model is evaluated using metrics such as accuracy, precision, recall, and F1 score. This step ensures that the model generalizes well to new, unseen data.
Fine-Tuning and Hyperparameter Optimization
To enhance performance, models undergo fine-tuning where hyperparameters are adjusted. Techniques like grid search or random search aid in finding optimal hyperparameter values.
Generalization and Overfitting
Ensuring that the model generalizes well to new data is crucial. Techniques like regularization and cross-validation help prevent overfitting, where the model becomes too specific to the training data.
Types of Machine Learning Algorithms
Supervised Learning Algorithms
1. Linear Regression: Predicting a continuous output based on input features.
2. Logistic Regression: Classifying data into discrete classes.
3. Support Vector Machines: Finding a hyperplane that best separates data into classes.
4. Decision Trees: Hierarchical structures to make decisions based on input features.
5. Random Forests: Ensembles of decision trees for improved performance.
Unsupervised Learning Algorithms
1. Clustering Algorithms: Grouping similar data points together (k-means, hierarchical clustering).
2. Dimensionality Reduction: Reducing the number of features while retaining essential information (PCA, t-SNE).
3. Anomaly Detection: Identifying rare instances or outliers in the data.
Reinforcement Learning Algorithms
1. Markov Decision Processes (MDPs): Mathematical models for decision-making in a dynamic environment.
2. Q-learning: Learning optimal actions in a Markov Decision Process.
3. Policy Gradients: Directly optimizing the policy of an agent.
Deep Learning
1. Artificial Neural Networks (ANNs): Mimic the structure and function of the human brain.
2. Convolutional Neural Networks (CNNs): Specialized for image-related tasks like object detection and recognition.
3. Recurrent Neural Networks (RNNs): Suitable for sequence data such as time series or natural language.
Ensembles and Boosting
1. Bagging: Building multiple models independently and combining their predictions.
2. Boosting: Building models sequentially, with each model correcting errors made by the previous ones.
3. Stacking: Combining the predictions of multiple models using another model.
Applications of Machine Learning
Natural Language Processing (NLP)
1. Sentiment Analysis: Determining the sentiment behind text data.
2. Text Classification: Categorizing text into predefined categories.
3. Language Translation: Translating text from one language to another.
4. Chatbots: Intelligent conversational agents.
Computer Vision
Object Detection: Identifying and locating objects in images or videos.
Image Recognition: Classifying images into predefined categories.
Facial Recognition: Identifying and verifying individuals based on facial features.
Autonomous Vehicles: ML algorithms for self-driving cars.
Recommender Systems
1. Collaborative Filtering: Recommending items based on user preferences and similarities.
2. Content-Based Filtering: Recommending items based on the attributes of the items and user preferences.
Fraud Detection
1. Identifying Fraudulent Patterns: ML algorithms analyze transaction data to detect unusual patterns.
2. Cybersecurity: ML in identifying and preventing cybersecurity threats.
Healthcare
1. Disease Diagnosis: ML aids in diagnosing diseases from medical data.
2. Personalized Medicine: Tailoring medical treatments based on individual patient data.
3. Drug Discovery: ML accelerates the drug discovery process.
Finance
1. Credit Scoring: Assessing creditworthiness of individuals using ML models.
2. Fraud Detection in Finance: Identifying fraudulent activities in financial transactions.
3. Algorithmic Trading: ML algorithms for automated trading decisions.
4. Risk Assessment: Evaluating financial risks using predictive models.
The Impact and Future of Machine Learning
Machine Learning has sparked a revolution in how computers understand and interact with data. Its applications span across diverse industries, enhancing efficiency, accuracy, and decision-making processes. As we progress into the future, the potential for Machine Learning is limitless.
Harnessing the Power of Data
Machine Learning thrives on data. The more high-quality data available, the better the models can learn and adapt. The ongoing growth of digital ecosystems and the advent of the Internet of Things (IoT) continue to generate vast amounts of data, providing fertile ground for ML applications.
Automation and Innovation
The ability of Machine Learning models to automate complex tasks is reshaping industries. Automation not only improves efficiency but also enables organizations to focus on innovation and creativity. Tasks that were once labor-intensive and time-consuming can now be accomplished swiftly and accurately through ML-driven automation.
Ethical Considerations
As Machine Learning permeates various aspects of our lives, ethical considerations become paramount. Issues such as bias in algorithms, privacy concerns, and the ethical use of AI technologies need careful consideration. Striking a balance between technological advancement and ethical responsibility is crucial for the responsible deployment of Machine Learning systems.
Advancements in Deep Learning
Deep Learning, a subset of Machine Learning, continues to advance rapidly. Breakthroughs in neural network architectures, training methodologies, and hardware acceleration contribute to the evolution of deep learning models. This progress has led to unprecedented achievements in tasks like natural language understanding, image generation, and playing complex games.
Interdisciplinary Collaboration
Machine Learning is not confined to computer science; it extends its reach into various disciplines. Collaborations between data scientists, domain experts, ethicists, and policymakers are becoming increasingly important. Interdisciplinary collaboration ensures that ML solutions align with real-world needs and address potential societal challenges.
Democratization of Machine Learning
The accessibility of ML tools and frameworks has increased, leading to the democratization of Machine Learning. This means that individuals with diverse backgrounds and expertise levels can engage in ML projects. Cloud-based platforms, open-source libraries, and user-friendly interfaces contribute to making Machine Learning more inclusive and approachable.
Challenges and Opportunities
While Machine Learning holds immense potential, it also presents challenges. Data quality, interpretability of complex models, and the need for large datasets are ongoing concerns. Moreover, ensuring fairness and transparency in ML algorithms poses challenges in applications like hiring, finance, and criminal justice.
Despite challenges, the opportunities presented by Machine Learning are vast. From personalized healthcare to optimized logistics, ML applications continue to reshape industries and create new possibilities.
NB: Machine Learning stands as a transformative force, shaping the future of technology and innovation. Its ability to learn from data, make predictions, and adapt to changing circumstances has propelled it to the forefront of artificial intelligence. Understanding the intricate web of concepts, paradigms, and applications in Machine Learning equips us with the knowledge to navigate this dynamic field.
As we move forward, the collaboration between human intelligence and machine learning algorithms will become increasingly integral to solving complex challenges. Ethical considerations, responsible deployment, and interdisciplinary collaboration will guide the evolution of Machine Learning, ensuring its positive impact on society.
Machine Learning is not just a technological tool; it is a catalyst for progress, ushering in an era where intelligent systems augment human capabilities, driving innovation and improving the quality of life across diverse domains. The journey into the world of Machine Learning is a continuous exploration, as its potential unfolds in ways that shape the technological landscape of tomorrow.