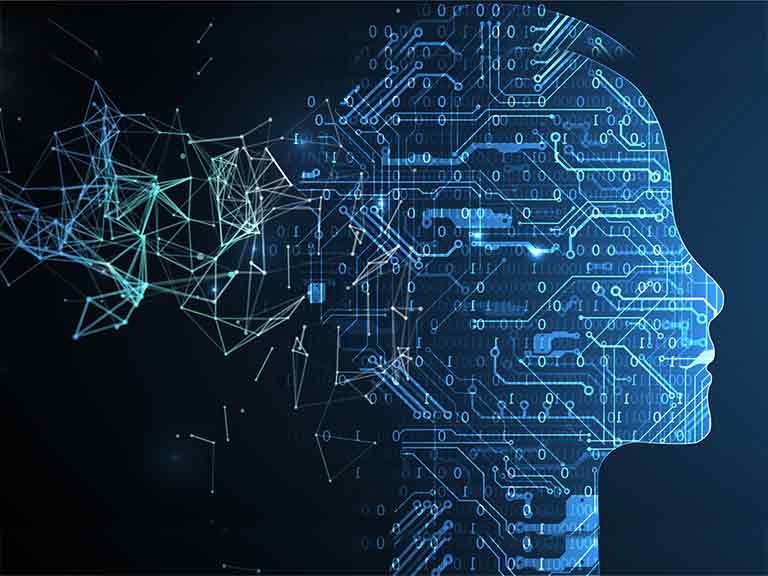
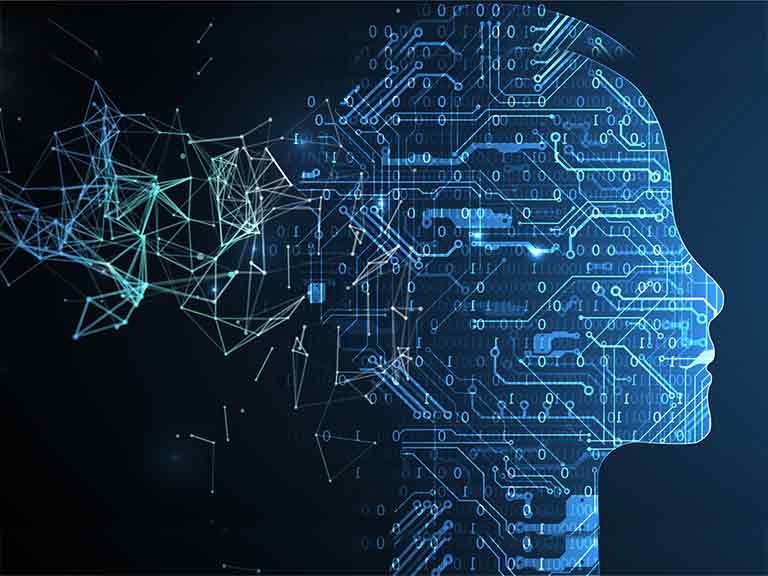
In the vast realm of artificial intelligence (AI) and deep learning, the journey from foundational principles to groundbreaking innovations has been nothing short of transformative. Central to this evolution are advancements in deep learning architectures, with Transformers and efficient transformer variants standing as luminous milestones. This article embarks on a comprehensive exploration of these advancements, elucidating their impact, underlying mechanisms, challenges, and future prospects.
The Rise of Transformers: A Paradigm Shift in NLP
The landscape of Natural Language Processing (NLP) has been marked by several milestones, but few have been as transformative as the introduction of the Transformer architecture. Unveiled by Google’s team of researchers in 2017, the Transformer model swiftly emerged as a beacon of innovation, reshaping the contours of sequence transduction and language modeling.
Attention Mechanism: The Heart of Transformers
Central to the Transformer’s groundbreaking capabilities is the attention mechanism. Drawing inspiration from human cognitive processes, this computational paradigm transcended the limitations of conventional neural network architectures, such as recurrent neural networks (RNNs) and convolutional neural networks (CNNs). While RNNs and CNNs predominantly operate in a sequential or hierarchical fashion, the attention mechanism empowers the Transformer model with the ability to dynamically weigh the relevance of different segments within input sequences.
This dynamic focus on salient information revolutionized context understanding in NLP. By discerning and prioritizing pertinent elements during sequence processing, the attention mechanism facilitated the creation of more nuanced, contextually rich, and accurate representations of language. This paradigm shift laid the foundation for a new era in NLP, characterized by enhanced model interpretability, adaptability, and performance across diverse tasks.
Revolutionizing NLP Tasks
The advent of the Transformer architecture catalyzed a wave of innovations and breakthroughs across the NLP landscape, spanning various domains and applications.
Language Understanding
The transformative impact of the Transformer model was palpably evident in the realm of language understanding. Models such as BERT (Bidirectional Encoder Representations from Transformers) heralded a new era of linguistic analysis, exhibiting unparalleled prowess in tasks ranging from text classification and sentiment analysis to named entity recognition. BERT’s bidirectional processing capabilities, enabled by the Transformer architecture, facilitated a holistic understanding of textual content, setting new benchmarks in language understanding and establishing new frontiers for AI-driven linguistic analysis.
Language Generation
Beyond understanding, the Transformer architecture showcased remarkable generative capabilities, epitomized by models like GPT (Generative Pre-trained Transformer). GPT’s ability to generate coherent, contextually relevant text heralded a paradigm shift in content creation and dialogue systems. From crafting engaging narratives and generating creative content to facilitating interactive dialogue systems, GPT and its variants leveraged the power of the Transformer architecture to unlock unprecedented possibilities in language generation, transcending traditional boundaries and redefining the contours of AI-driven content creation.
Language Translation
The Transformer architecture’s impact reverberated across global linguistic landscapes, most notably in the domain of machine translation. Transformer-based models, exemplified by Google’s Neural Machine Translation (GNMT) system, demonstrated unparalleled proficiency in bridging linguistic barriers, facilitating seamless communication across diverse languages and cultures. By leveraging the Transformer architecture’s inherent capabilities for contextual understanding and sequence transduction, GNMT and similar systems transcended traditional limitations, ushering in a new era of machine translation characterized by enhanced accuracy, fluency, and linguistic fidelity.
Efficient Transformers: Bridging Scalability and Performance
The advent of the Transformer architecture heralded a new era in Natural Language Processing (NLP), propelling the field into realms previously deemed unattainable. However, as the Transformer models gained prominence and adoption, a pressing challenge emerged: the computational demands associated with processing longer sequences. In response to these challenges, the global research community rallied behind a common goal – the development of efficient transformer variants capable of reconciling scalability with performance.
Sparse Transformers: Optimizing Computation
Sparse Transformers emerged as a beacon of innovation, offering a groundbreaking solution to the computational inefficiencies plaguing traditional transformer architectures. At the heart of Sparse Transformers lies a paradigm shift in attention mechanism design. Rather than uniformly attending to all input tokens, Sparse Transformers employ sparse attention patterns, selectively focusing on a subset of input tokens.
This strategic focus not only alleviates the computational burden associated with self-attention mechanisms but also unlocks new horizons in model efficiency and scalability. By judiciously allocating computational resources to salient segments of input sequences, Sparse Transformers transcend traditional limitations, accelerating both model training and inference processes.
Furthermore, the advent of Sparse Transformers ushered in a new era of capability in NLP, particularly for tasks demanding extensive context understanding. Applications such as document summarization and long-form content generation witnessed remarkable advancements, with Sparse Transformers demonstrating unparalleled proficiency in handling lengthy sequences, preserving contextual coherence, and generating coherent and concise summaries and narratives.
Longformer: Enabling Scalable Sequence Processing
Building upon the foundation laid by Sparse Transformers, Longformer introduced a revolutionary approach to sequence processing, specifically tailored to address the challenges of handling extended sequences. Longformer’s architecture embodies a harmonious blend of local and global attention mechanisms, designed to strike an optimal balance between context coverage and computational efficiency.
By judiciously integrating local attention mechanisms, which focus on neighboring tokens, with global attention mechanisms, capable of capturing broader contextual relationships, Longformer transcends the limitations of traditional transformers, enabling efficient and effective processing of extensive textual data.
The distinctive design philosophy of Longformer facilitates seamless context aggregation across lengthy documents, empowering the model to capture intricate relationships, dependencies, and nuances within extensive textual data. This enhanced contextual understanding underpins Longformer’s remarkable performance across diverse NLP tasks, from document analysis and summarization to content generation and knowledge discovery.
Challenges and Considerations
The transformative impact of transformer architectures on the landscape of deep learning is undeniable. However, as with any groundbreaking innovation, the proliferation of transformers and their variants also brings forth a myriad of challenges and considerations that warrant meticulous scrutiny and innovative solutions.
Model Complexity: Navigating the Labyrinth of Computational Demands
One of the most salient challenges associated with transformer architectures is their intrinsic complexity. Characterized by intricate network designs, multi-layered structures, and sophisticated attention mechanisms, transformers necessitate a formidable computational infrastructure to realize their full potential. This elevated computational demand poses a significant barrier, particularly for deployment on resource-constrained devices, such as edge devices, mobile platforms, and IoT (Internet of Things) environments, where computational resources are inherently limited.
The pursuit of efficient transformer variants, such as Sparse Transformers and Longformer, represents a concerted effort to mitigate these challenges. However, achieving optimal performance on constrained resources remains an ongoing endeavor, requiring innovative strategies, model optimization techniques, and advancements in hardware acceleration to navigate the intricate labyrinth of computational demands inherent to transformer architectures.
Scalability: Bridging the Gulf Between Efficiency and Extensibility
Another formidable challenge confronting the broader adoption of transformer architectures pertains to scalability. While efficient transformer variants have made remarkable strides in reconciling performance with computational efficiency, the task of developing models capable of processing exceedingly long sequences without sacrificing efficiency remains a formidable challenge.
The ability to handle extended sequences is paramount for numerous real-world applications, such as document analysis, content generation, and long-form text processing. Addressing this challenge necessitates a multifaceted approach, encompassing algorithmic innovations, architectural refinements, and advancements in parallel computing paradigms, to bridge the gulf between efficiency and extensibility, unlocking new horizons in sequence processing and enabling transformative advancements across diverse domains and applications.
Generalization and Robustness: Charting Uncharted Territories
Ensuring the generalization and robustness of transformer models across diverse datasets and real-world scenarios constitutes another pivotal challenge that underscores the complexities inherent to transformer architectures. While transformers have demonstrated remarkable performance across a myriad of tasks and benchmarks, their true efficacy is contingent upon their ability to generalize across unseen data distributions, adapt to dynamic environments, and exhibit resilience against adversarial perturbations and anomalies.
Addressing this multifaceted challenge necessitates a holistic approach, encompassing innovative training techniques, robust optimization strategies, comprehensive evaluation methodologies, and rigorous validation protocols, to foster the development of transformer models that transcend traditional boundaries, exhibit unparalleled robustness, and empower AI-driven systems to navigate the complexities of real-world scenarios with confidence, reliability, and efficacy.
Future Directions and Opportunities
The trajectory of advancements in deep learning and Natural Language Processing (NLP) continues to chart unprecedented territories, fueled by relentless innovation, collaborative research endeavors, and the inexhaustible quest to push the boundaries of what’s possible. As we stand on the precipice of a new era in AI, the horizon brims with promise, heralding transformative developments, groundbreaking innovations, and a myriad of opportunities that promise to reshape the landscape of AI-driven technologies and applications.
Hybrid Architectures: Bridging Neural Paradigms for Enhanced Performance
At the forefront of future directions in transformer architectures lies the evolution and proliferation of hybrid architectures. The nascent endeavors in this domain seek to amalgamate the unparalleled strengths of transformers with other neural network paradigms, fostering synergies that transcend the limitations of individual architectures and culminate in models characterized by enhanced performance, versatility, and adaptability.
The potential of hybrid architectures extends beyond mere amalgamation, paving the way for novel neural network configurations that synergistically combine the attention mechanisms of transformers with the hierarchical processing capabilities of convolutional neural networks (CNNs) and the sequential modeling prowess of recurrent neural networks (RNNs). This confluence of neural paradigms promises to unlock new horizons in AI, enabling the development of models capable of harnessing the complementary strengths of diverse architectures, fostering innovation, and driving advancements across a myriad of applications, domains, and research frontiers.
Efficient Inference: Pioneering the Next Frontier in AI Deployment
As the capabilities of transformer architectures burgeon, the imperative to optimize inference processes and streamline model deployment becomes increasingly salient. The future heralds a plethora of innovations in model optimization techniques, algorithmic refinements, and hardware acceleration paradigms, poised to catalyze advancements in efficient inference and pave the way for the widespread adoption of transformer-based models across diverse platforms, environments, and applications.
Innovative strategies, such as quantization, pruning, and model distillation, are anticipated to mitigate computational overheads, enhance inference speed, and facilitate seamless deployment of transformer architectures on edge devices, IoT environments, and real-time applications. These advancements in efficient inference are not merely technological milestones but represent a paradigm shift in AI deployment, enabling transformative applications, fostering ubiquitous intelligence, and empowering AI-driven systems to operate with unparalleled efficiency, responsiveness, and agility across diverse operational landscapes.
Multimodal Transformers: Unleashing the Power of Multimodal Learning
The integration of transformer architectures with multimodal learning frameworks emerges as a pivotal frontier, poised to unlock new dimensions of AI capabilities and redefine the boundaries of content processing, generation, and interaction. Multimodal transformers, characterized by their ability to process and generate content across diverse modalities, such as text, images, and audio, herald a new era of AI-driven innovation, facilitating the development of models capable of synthesizing insights, fostering contextual understanding, and enabling holistic interactions across multimodal data streams.
The convergence of transformer architectures with multimodal learning paradigms promises to foster the development of AI systems that transcend traditional boundaries, exhibit enhanced contextual awareness, and empower users to interact, engage, and immerse themselves in AI-driven experiences that seamlessly integrate diverse data modalities, enrich human-machine interactions, and drive transformative advancements across a myriad of domains, applications, and research frontiers.
NB: The continued advancements in Transformers and efficient transformer variants epitomize the relentless pursuit of excellence and innovation in the field of deep learning. As we harness the transformative power of these architectures, we embark on a journey towards unraveling the mysteries of human language and cognition, paving the way for a future where AI transcends boundaries, enriches experiences, and empowers humanity to forge new frontiers of knowledge and discovery.
Can you be more specific about the content of your article? After reading it, I still have some doubts. Hope you can help me.
We will work towards that sir. Thank you for your views.